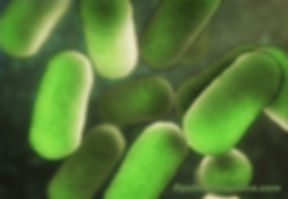
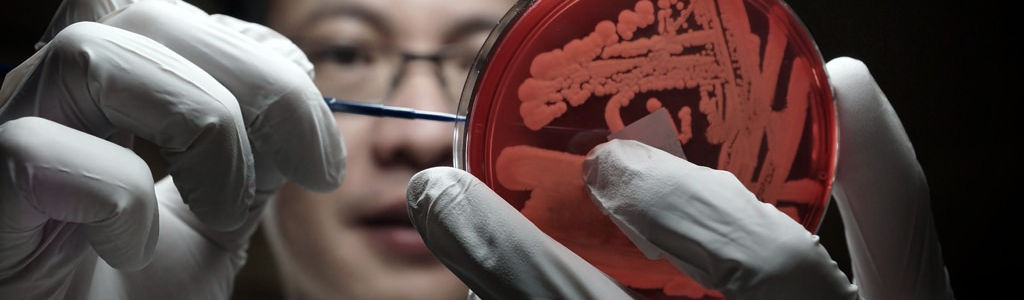
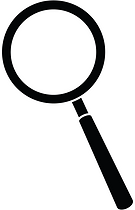
Epidemiology: Disease Detectives
Causal Inference
One of the biggest parts in epidemiology is learning how to analyze data, doing calculations, and analyzing graphs in order to determine the source or cause of disease. However, it is also necessary to understand one more concept, the Bradford Hill Criteria, before getting into the more technical or mathematical topics.
Bradford Hill Criteria
Strength of the Association
“According to Hill, the stronger the association between a risk factor and outcome, the more likely the relationship is to be causal.”The relationship must be clear and the risk estimate must be high.
Consistency of Findings
“Have the same findings must be observed among different populations, in different study designs and different times.”
Specificity of the association
“There must be a one to one relationship between cause and outcome.”In other words, a single cause must cause a single effect.
Temporal Sequence of Association
“Exposure must precede outcome.”
Biological Gradient
"Changes in disease rates should follow from corresponding changes in exposure (does-response).” Dose response is the relationship in which an increasing amount of exposure will increase the risk of obatianing the disease.
Plausiblity
“Does it make sense?”
Coherence
“Does the relationship agree with the current knowledge of the natural history/biology of the disease?”
Experimental evidence
“Does the removal of the exposure alter the frequency of the outcome?”
Reasoning by Analogy
“Is the observed association supported by simalar associations?”
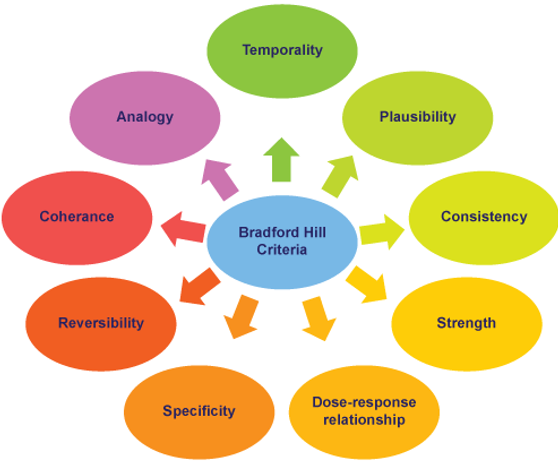
Simple Calculations
Epidemiologists are often given data and are asked to interpret them.
Imagine the following scenario:
"An outbreak of severe stomach illness occured following a student party. Disease detectives took up the challenge to investigae and obtained the following data of association of specific food eaten at the party and having the illness." [Citation 55]
The following data table corresponds with the scenario:
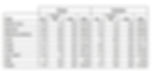
Calculating Relative Risk (RR)
Relative risk (RR) is a ratio of the risk of the disease among people exposed and risk of people who were unexposed. It generally helps communicates how many times or how likely someone is to develop a disease. This calculation is usually done in: cohort studies and randomized control trials. [Citation 56]
The formula is:

As an example, lets calculate the relative risk for the ice cream. Before starting, when calculating relative risk, it is extremely helpful to conduct a table.
Conduct a table like the following:

However, replace it for the data that applies to baked ham.
Replace the variables in the table with the following numbers:
A=43 (the number of people exposed and got sick)
B=11 ( the number of people exposed and not sick)
C=13 (the number of people not exposed and sick)
D=18 (the number of people not exposed and not sick)
A+B=43+11=54
C+D=13+18=31
A+C=43+13=56
B+D=11+18=29
A+B+C+D=85
Now that the table is filled out, it's easier to plug the numbers into the formula.
RR= (43/54) / (13/31) = 1.9
This value of 1.9 would communicate to disease detectives that ice cream has a relative risk of 1.9, which means that those who ate ice cream were 1.9 more likely to develop the disease. It is the value that indicates the risk of developing the illness due to that action or risk behavior. (The relative risk can also be calculated and compared for the other food items.)

Calculating the Odds Ratio (OR)
The odds ratio (OR) is the "ratio of the probability of occurence of an event to that of non-occurence" and tells epidemiologists how variables are "associated with the outcome compared to other variables". [Citation 56, 59]
The odds ratio is caculated using a simalar table. However the formula is:
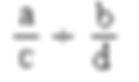
It is important to keep in mind--for future reference--that the odds ratio is used in case-control studies and not relative risk. Because in this type of study, "we already know what the outcome is and we separate groups...our objective in such studies is to identify risk factors that are strongly associated with one group than the other". This explains why one probability is compared to another probability in the odds ratio.